引用本文: | 赵彤,李怀璐,王旭,张伟伟.面向战斗机尾旋仿真的增量叠加神经网络模型[J].哈尔滨工业大学学报,2025,57(4):10.DOI:10.11918/202410071 |
| ZHAO Tong,LI Huailu,WANG Xu,ZHANG Weiwei.Incremental superposition neural network model for fighter jet spin simulation[J].Journal of Harbin Institute of Technology,2025,57(4):10.DOI:10.11918/202410071 |
|
|
|
本文已被:浏览 109次 下载 93次 |
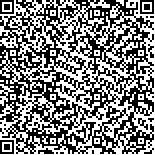 码上扫一扫! |
|
面向战斗机尾旋仿真的增量叠加神经网络模型 |
赵彤1,3,李怀璐2,3,王旭2,张伟伟2
|
(1.南京航空航天大学 航空学院,南京 210016; 2.西北工业大学 航空学院,西安 710072; 3.中国航空工业集团公司 沈阳飞机设计研究所,沈阳 110035)
|
|
摘要: |
为提升战斗机尾旋过程中的气动力预测能力,改善稳定尾旋运动的仿真精度,结合深度神经网络强大的函数拟合能力,提出了一种新型神经网络模型,实现对尾旋非定常气动力的准确建模,并通过尾旋耦合仿真开展高精度的尾旋姿态预测。以战斗机失速尾旋下的气动特性为研究对象,首先,利用神经网络模型对立式风洞试验中的非定常气动力矩进行高精度建模。其次,基于神经网络模型特点与传统气动数据库构造方式,提出了增量叠加神经网络模型,将气动数据库的舵效增量引入神经网络,实现了变舵面下的尾旋非定常气动力矩的高精度建模。最后,将得到模型与尾旋运动学方程耦合,开展稳定尾旋仿真与尾旋特征预测。研究结果表明,所提出的模型能够很好地刻画不同舵面组合下尾旋气动力变化,与传统气动数据库相比气动力矩预测误差降低77%,利用该模型能够实现对飞机稳定尾旋特性的高精度预测,其中稳定尾旋周期预测相对误差降低34%,验证了机器学习方法在飞机复杂动力学仿真中的工程有效性。 |
关键词: 立式风洞 尾旋仿真 神经网络 飞行动力学 非定常气动力 |
DOI:10.11918/202410071 |
分类号:V211 |
文献标识码:A |
基金项目:国家自然科学基金(U2441211) |
|
Incremental superposition neural network model for fighter jet spin simulation |
ZHAO Tong1,3,LI Huailu2,3,WANG Xu2,ZHANG Weiwei2
|
(1.College of Aerospace Engineering, Nanjing University of Aeronautics and Astronautics, Nanjing 210016, China; 2.School of Aeronautics, Northwestern Polytechnical University, Xi’an 710072, China; 3.Shenyang Aircraft Design and Research Institute, Aviation Industry Corporation of China, Ltd., Shenyang 110035, China)
|
Abstract: |
To improve the aerodynamic prediction capability during fighter jet spin maneuvers and enhance the simulation accuracy of stable spin motion, a novel neural network model is proposed, leveraging the powerful function approximation capabilities of deep neural networks. This model enables accurate modeling of the unsteady aerodynamic forces during spin maneuvers and achieves high-precision spin attitude prediction through spin-coupled simulation. Focusing on the aerodynamic characteristics of fighter jets in post-stall spin, this study first utilizes the neural network model to achieve high-precision modeling of the unsteady aerodynamic moments observed in vertical wind tunnel tests. Secondly, based on the features of the neural network model and traditional aerodynamic database construction methods, an incremental superposition neural network model is proposed. This model incorporates control surface deflection increments from aerodynamic databases into the neural network, enabling high-precision modeling of unsteady aerodynamic moments under varying control surface configurations. Finally, the resulting model is then coupled with the spin motion equations to conduct stable spin simulations and spin characteristic predictions. The research results indicate that the proposed model effectively captures variations in spin aerodynamics under different control surface combinations. Compared to traditional aerodynamic databases, the aerodynamic moment prediction error is reduced by 77%. Using this model enables high-precision prediction of stable spin characteristics, with the relative error in stable spin period prediction reduced by 34%, demonstrating the engineering effectiveness of machine learning methods in simulating complex aircraft dynamics. |
Key words: vertical wind tunnel spin simulation neural network flight dynamics unsteady aerodynamics |
|
|
|
|