引用本文: | 吴丹,杨基先,李昂,邢洁,马放,魏薇.基于BP神经网络的产絮菌发酵参数的优化[J].哈尔滨工业大学学报,2013,45(10):30.DOI:10.11918/j.issn.0367-6234.2013.10.006 |
| WU Dan,YANG Jixian,LI Ang,XING Jie,MA Fang,WEI Wei.Optimization of flocculant-producing bacteria fermentation parameters by BP neural network[J].Journal of Harbin Institute of Technology,2013,45(10):30.DOI:10.11918/j.issn.0367-6234.2013.10.006 |
|
|
|
本文已被:浏览 2253次 下载 1097次 |
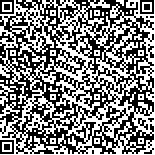 码上扫一扫! |
|
基于BP神经网络的产絮菌发酵参数的优化 |
吴丹1,2, 杨基先1,2, 李昂1,2, 邢洁1,2, 马放1,2, 魏薇1,2
|
(1. 哈尔滨工业大学 城市水资源与水环境国家重点实验室, 150090 哈尔滨; 2. 哈尔滨工业大学 市政环境工程学院, 150090 哈尔滨)
|
|
摘要: |
为优化对产絮菌克雷伯氏菌(Klebsiella. sp.)的发酵参数,首先用正交实验量化絮凝菌发酵参数对絮凝率和产率的影响度,确定温度、摇床转数、pH 3个影响度较高的发酵参数作为神经网络的输入,絮凝率和产率为输出,同时设计了训练样本.经过反复训练,建立了准确度高、误差小的预测模型,并利用该模型实现发酵参数双指标的全局优化,其最优发酵条件为温度33 ℃、摇床转数141 r/min、pH 7.90,经验证此条件下实际絮凝率和产率分别为92.67%和2.180 9 g/L,絮凝率提高了4.08%,产率提高了14.36%,使生物絮凝剂产量低的问题得以初步改善.利用所建立模型预测生物絮凝菌F+在发酵罐中的发酵过程,仿真误差较小. |
关键词: 产絮凝 发酵 BP神经网络 |
DOI:10.11918/j.issn.0367-6234.2013.10.006 |
分类号: |
基金项目:国家高技术研究发展计划项目(863 计划)(2009AA062906); 国家创新研究群体科学基金项目(51121062); 城市水资源与水环境国家重点实验室(哈尔滨工业大学)自主课题(2010TX3,0DX09). |
|
Optimization of flocculant-producing bacteria fermentation parameters by BP neural network |
WU Dan1,2, YANG Jixian1,2, LI Ang1,2, XING Jie1,2, MA Fang1,2, WEI Wei1,2
|
(1. State Key Lab of Urban Water Resource and Environment, Harbin Institute of Technology, 150090 Harbin, China; 2. School of Municipal and Environmental Engineering, Harbin Institute of Technology, 150090 Harbin, China)
|
Abstract: |
To optimize the fermentation parameter of Klebsiella. sp, orthogonal experiments were used to quantify the degree of the fermentation parameters of flocculant-producing bacteria MFX on the flocculation rate and the yield, which indentified temperature, shaking revolution and pH value as the input of neural network, flocculation rate and yield as the output of neural network, and then a training sample was designed. After repeat training, a prediction model with high accuracy and small error was established by which the optimum fermentation conditions: the temperature of 33 ℃, the stirring speed at 141 r/min and pH value of 7.90, were obtained. Meanwhile, the actual flocculation rate and the yields were 92.67% and 2.180 9 g/L, respectively. The flocculation rate increased 4.08%, and the yields of bio-flocculant increased 14.36%, which improved the yield of bio-flocculant. The model was used to predict the fermentation process of flocculant-producing bacteria F+in the fermenter, the simulation error was small, which was the basis for the prediction and control of industrial fermentation process. |
Key words: flocculant-producing bacteria fermentation BP neural network |
|
|
|
|