引用本文: | 段喜萍,刘家锋,王建华,唐降龙.一种语义级文本协同图像识别方法[J].哈尔滨工业大学学报,2014,46(3):49.DOI:10.11918/j.issn.0367-6234.2014.03.009 |
| DUAN Xiping,LIU Jiafeng,WANG Jianhua,TANG Xianglong.A collaborative image recognition method based on semantic level of text[J].Journal of Harbin Institute of Technology,2014,46(3):49.DOI:10.11918/j.issn.0367-6234.2014.03.009 |
|
本文已被:浏览 1870次 下载 1367次 |
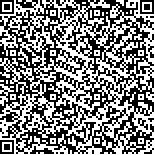 码上扫一扫! |
|
一种语义级文本协同图像识别方法 |
段喜萍1,2,3,刘家锋1,王建华2,3,唐降龙1
|
(1.哈尔滨工业大学 计算机科学与技术学院,150001 哈尔滨; 2. 哈尔滨师范大学 计算机科学与信息工程学院, 150025 哈尔滨; 3. 黑龙江省高校智能教育与信息工程重点实验室, 150025 哈尔滨)
|
|
摘要: |
为解决单纯依赖图像低级视觉模态信息进行图像识别准率低的问题. 考虑到许多图像中存在文本信息,提出了利用图像中的文本信息辅助图像识别的语义级文本协同图像识别方法. 该方法通过文本定位方法定位到图像中的文本块,对其进行分割、二值化、提取特征等处理;然后获取语义,提取图像底层视觉信息,计算两模态的相关性,从而得到协同后验概率; 最后,得到联合后验概率,并取其中最大联合后验概率对图像进行识别. 在自建体育视频帧数据库中,通过与以朴素贝叶斯为代表的单模态方法进行比较,方法在3种不同视觉特征下均具有更高的准确率. 实验结果表明,文本协同方法能够有效辅助图像识别,具有更好的识别性能. |
关键词: 文本定位 图像识别 多模态 |
DOI:10.11918/j.issn.0367-6234.2014.03.009 |
分类号: |
基金项目:国家自然科学基金资助项目 (7,2). |
|
A collaborative image recognition method based on semantic level of text |
DUAN Xiping1,2,3, LIU Jiafeng1, WANG Jianhua2,3, TANG Xianglong1
|
(1.School of Computer Science and Technology, Harbin Institute of Technology, 150001 Harbin, China; 2.Computer Science and Information Engineering College, Harbin Normal University, 150025 Harbin, China; 3. Heilongjiang Provincial Key Laboratory of Intelligence Education and Information Engineering, 150025 Harbin, China )
|
Abstract: |
To solve the problem that singular-modal image recognition using only the low-level visual features has low accuracy, considering that many images have embedded-in textual information, a collaborative method using the embedded-in text to aid the recognition of images is proposed. The method includes three steps. Firstly, after localization, segmentation, binarization and feature extraction, semantics of text is gotten. Secondly, the collaborative posterior probability is calculated by extracting visual features of images and counting correlation of visual and textual modals. At last, for each class of images, the joint posterior probability is calculated using the previous two items. A new image is recognized to the class with maximal joint posterior probability. Experiments on the self-built data set of sports video frames showed that the proposed method performed better than the singular-modal method on three different visual features and had higher accuracy. |
Key words: text localization image recognition multi-modal |