引用本文: | 田秀娟,于德新,邢雪,商强,王树兴.交叉口短时流量CEEMDAN-PE-OSELM预测模型[J].哈尔滨工业大学学报,2018,50(3):83.DOI:10.11918/j.issn.0367-6234.201703102 |
| TIAN Xiujuan,YU Dexin,XING Xue,SHANG Qiang,WANG Shuxing.Prediction model of CEEMDAN PE OSELM for intersections short term traffic flow[J].Journal of Harbin Institute of Technology,2018,50(3):83.DOI:10.11918/j.issn.0367-6234.201703102 |
|
本文已被:浏览 1306次 下载 893次 |
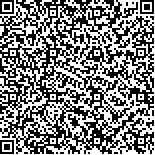 码上扫一扫! |
|
交叉口短时流量CEEMDAN-PE-OSELM预测模型 |
田秀娟1,于德新1,2,邢雪3,商强1,王树兴4
|
(1.吉林大学 交通学院,长春 130022; 2. 吉林省道路交通重点实验室,长春 130022; 3.吉林化工学院 信息与控制工程学院,吉林 吉林132022; 4. 山东高速股份有限公司,济南 250000)
|
|
摘要: |
为提高交叉口短时交通流预测精度,以历史交通流量数据为基础,提出一种基于自适应噪声完整集成经验模态分解(complete ensemble empirical mode decomposition with adaptive noise,CEEMDAN)-排列熵(permutation entropy,PE)-在线序贯极限学习机(online sequential extreme learning machine,OSELM)组合预测模型(CEEMDAN-PE-OSELM).首先对交通流历史时间序列数据进行CEEMDAN分解,得到多个本征模态函数(intrinsic mode function,IMF)分量;通过PE算法对IMF分量进行重组,形成具有复杂度差异的重组子序列.然后,分别构建重组子序列OSELM预测模型,将预测结果相加得到最终预测流量.最后选取一实际交叉口,进行模型验证分析.结果表明:CEEMDAN-PE-OSELM模型的MAE、MAPE和MSE的值均低于其他模型,预测误差最小;EC值为0.963,高于ARIMA模型的EC值(0.898),最接近于1,预测精度最高,稳定性最好.就同一预测模型而言,经过CEEMDAN-PE处理的模型的各项误差明显降低,预测精度有所提高.
|
关键词: 时间序列 短时流量预测 组合预测 经验模态分解 极限学习机 |
DOI:10.11918/j.issn.0367-6234.201703102 |
分类号:U491 |
文献标识码:A |
基金项目:国家科技支撑计划项目(2014BAG03B03); 山东省省管企业科技创新项目(20122150251-1) |
|
Prediction model of CEEMDAN PE OSELM for intersections short term traffic flow |
TIAN Xiujuan1,YU Dexin1,2,XING Xue3,SHANG Qiang1,WANG Shuxing4
|
(1. College of Transportation, Jilin University, Changchun 130022, China; 2. Jilin Province Key Laboratory of Road Traffic, Changchun 130022, China; 3. College of Information and Control Engineering, Jilin Institute of Chemical Technology, Jilin 132022, Jilin, China;4. Shandong High-Speed Company Limited, Jinan 250000, China)
|
Abstract: |
To improve the prediction accuracy of intersection short-term traffic flow, a new CEEMDAN-PE-OSELM model is developed based on the complete ensemble empirical mode decomposition with adaptive noise(CEEMDAN), permutation entropy(PE) and online sequential extreme learning machine(OSELM). Firstly, traffic flow historical time series are decomposed by CEEMDAN algorithm. Secondly, PE algorithm is used to recombine the IMF components obtained by CEEMDAN, and a series of restructured subsequences can be obtained, which have a significant difference in terms of complexity. Then, the OSELM prediction models are proposed for each restructured subsequence respectively, and the final results are got by adding the prediction results. Finally, a typical intersection is verified the effect and performance of the hybrid prediction model. Results show that the values of MAE, MAPE and MSE of CEEMDAN-PE-OSELM prediction model are lower than other models, and get a minimal error. The EC value of the improved model is 0.963, which is higher than that of ARIMA model (0.898) and the most close to 1. The CEEMDAN-PE-OSELM prediction model has the highest precision and best stability, and the errors decrease obviously.
|
Key words: time series short-term traffic prediction hybrid prediction empirical mode decomposition extreme learning machine |