引用本文: | 周雅夫,刘邵勋,孙宵宵,黄立建,连静.车用动力电池全生命周期寿命预测方法[J].哈尔滨工业大学学报,2022,54(6):37.DOI:10.11918/202007052 |
| ZHOU Yafu,LIU Shaoxun,SUN Xiaoxiao,HUANG Lijian,LIAN Jing.Prediction method for full lifetime of vehicle power battery[J].Journal of Harbin Institute of Technology,2022,54(6):37.DOI:10.11918/202007052 |
|
|
|
本文已被:浏览 683次 下载 684次 |
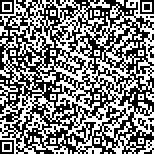 码上扫一扫! |
|
车用动力电池全生命周期寿命预测方法 |
周雅夫1,2,刘邵勋1,2,孙宵宵1,2,黄立建1,2,连静1,2
|
(1.工业装备结构分析国家重点实验室(大连理工大学),辽宁 大连 116024; 2.大连理工大学 运载工程与力学学部汽车工程学院,辽宁 大连 116024)
|
|
摘要: |
为准确量化车用动力电池老化程度,提升其行业利用率,实现电池的全生命周期剩余寿命(Remaining useful life, RUL)的精确预测,提出一种基于多系数模型的车用动力电池全生命周期寿命预测方法。该方法融合重组了传统的经验指数模型和改进后的多项式回归模型,重组后的模型能在实验数据分析的基础上追踪电池全生命周期内的寿命退化趋势。该方法采用粒子滤波(Particle filter, PF)思想在线调整模型参数,设计了针对动力电池不同状态,不同容量种类的算例预测电池的RUL,通过改进多项式回归模型,传统经验指数模型以及多系数模型的预测精度对比评估模型。实验结果表明:相较于经验指数模型和改进后的多项式回归模型,本文提出的多系数模型针对电池容量衰减具有更好的拟合能力;结合粒子滤波算法,该模型无论是对在役电池还是退役电池均具有高精度的寿命预测结果。该方法对不同容量的动力电池均能准确预测电池失效时间,在电池梯次利用行业具有一定的适用性。 |
关键词: 动力电池 寿命预测 粒子滤波 融合模型 容量衰减 |
DOI:10.11918/202007052 |
分类号:TM911 |
文献标识码:A |
基金项目:国家重点研发计划(2018YFE0105100) |
|
Prediction method for full lifetime of vehicle power battery |
ZHOU Yafu1,2,LIU Shaoxun1,2,SUN Xiaoxiao1,2,HUANG Lijian1,2,LIAN Jing1,2
|
(1.State Key Laboratory of Structural Analysis for Industrial Equipment (Dalian University of Technology), Dalian 116024, Liaoning, China; 2.School of Automotive Engineering, Faculty of Vehicle Engineering and Mechanics, Dalian University of Technology, Dalian 116024, Liaoning, China)
|
Abstract: |
A full lifetime capacity prediction method for vehicle power batteries was proposed, so as to accurately quantify the aging degree of automotive power batteries, improve the utilization rate of batteries, and achieve accurate prediction of the remaining useful life (RUL) in the whole life cycle of batteries. By integrating the traditional empirical exponential model and the improved polynomial regression model, the proposed method could track the degradation trend of battery life cycle based on the analysis of experimental data. The particle filter (PF) was adopted to adjust the model parameters online. Experiments were carried out to predict the RUL of power batteries with different states and capacities. The model was evaluated by comparing the prediction accuracy of different models. Experimental results show that the proposed model had a stronger ability in battery capacity attenuation tracking than that of the traditional empirical exponential model and the improved polynomial regression model. Combined with particle filter algorithm, the method achieved high-precision prediction results for both in-service and retired batteries. Besides, the method could accurately predict the failure time of power batteries with different capacities, which has a wide applicability in battery cascade utilization. |
Key words: power batteries life prediction particle filtering fusion model capacity attenuation |
|
|
|
|