引用本文: | 杨燕,张全君,梁皓博.混合注意力与多特征交互的去雾算法[J].哈尔滨工业大学学报,2024,56(9):56.DOI:10.11918/202404029 |
| YANG Yan,ZHANG Quanjun,LIANG Haobo.Dehazing algorithm based on mixed attention and multi-feature interaction[J].Journal of Harbin Institute of Technology,2024,56(9):56.DOI:10.11918/202404029 |
|
|
|
本文已被:浏览 703次 下载 1062次 |
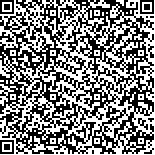 码上扫一扫! |
|
混合注意力与多特征交互的去雾算法 |
杨燕,张全君,梁皓博
|
(兰州交通大学 电子与信息工程学院,兰州 730070)
|
|
摘要: |
为解决目前深度学习去雾算法在处理非均匀雾天图像时无法有效利用多尺度特征,导致复原出的图像产生颜色失真、细节恢复不完整等问题,提出了混合注意力与多特征交互的图像去雾算法。首先,利用编码模块提取不同尺度的特征;其次,构造混合注意力模块,从全局角度对图像雾气进行感知,并利用通道注意力机制对不同雾浓度分配权重;然后,设计多特征交互模块,实现不同尺度特征间的信息交换,有效利用低分辨率特征中的语义信息,同时保留了高分辨率特征的空间细节与颜色信息,并利用门控融合模块聚合不同尺度的特征;最后,解码模块对融合后的特征进行重构,得到无雾图像。实验结果表明,运用本文提出的算法恢复的去雾图像不仅主观上颜色自然、细节清晰,而且在客观指标上也优于现有的主流算法。该研究结果可为深度学习去雾研究与应用提供新的方案。 |
关键词: 图像去雾 编解码器 混合注意力 多特征交互 门控融合 |
DOI:10.11918/202404029 |
分类号:TP391.41 |
文献标识码:A |
基金项目:国家自然科学基金(61561030);甘肃省高等学校产业支撑计划项目(2021CYZC-04);兰州交通大学教改项目(JG201928) |
|
Dehazing algorithm based on mixed attention and multi-feature interaction |
YANG Yan,ZHANG Quanjun,LIANG Haobo
|
(School of Electronic and Information Engineering, Lanzhou Jiaotong University, Lanzhou 730070, China)
|
Abstract: |
In order to solve the problems that the current deep learning dehazing algorithm being ineffective in using multi-scale features when processing non-uniform foggy images, resulting in color distortion and incomplete detail recovery of the restored images, this paper proposes an image dehazing algorithm with mixed attention and multi-feature interaction. Firstly, the coding module is used to extract features at different scales. Secondly, a mixed attention module is constructed to globallay perceive the image fog weights to different fog concentrations using channel attention mechanisms. Then, a multi-feature interaction module is designed to facilitate information exchange between features of different scales, effectively use the semantic information in low-resolution features, retain the spatial details and color information of high-resolution features, and use the gated fusion module to aggregate features of different scales. Finally, the decoding module reconstructs the fused features to obtain a fog-free image. Experimental results show that the dehazing images recovered by the proposed algorithm not only have natural colors and clear details subjectively, but also outperform the existing mainstream algorithms in objective indicators. These research findings offer novel approach for both research and application of deep learning-based image dehazing. |
Key words: image dehazing, codec mixed attention multi-feature interaction gated fusion |
|
|
|
|