引用本文: | 吴鸿敏,张国英,管贻生,JUAN Rojas.基于多模态时间序列建模的机器人安全监控[J].哈尔滨工业大学学报,2020,52(1):126.DOI:10.11918/201809143 |
| WU Hongmin,ZHANG Guoying,GUAN Yisheng,JUAN Rojas.Multimodal time series modeling-based robot safety surveillance[J].Journal of Harbin Institute of Technology,2020,52(1):126.DOI:10.11918/201809143 |
|
|
|
本文已被:浏览 1761次 下载 1426次 |
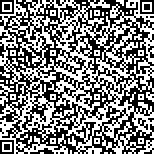 码上扫一扫! |
|
基于多模态时间序列建模的机器人安全监控 |
吴鸿敏1,2,张国英1,管贻生1,JUAN Rojas1
|
(1.广东工业大学 机电工程学院,广州 510006; 2.广东省智能制造研究所 广东省现代控制技术重点实验室, 广州 510070)
|
|
摘要: |
针对多模态时间序列建模的机器人安全监控问题,基于多模态粘性层级狄利克雷过程隐马尔可夫模型(MD-sHDPHMM)提出了一种能准确、可靠、快速、通用地实现机器人执行过程识别与异常事件监测的方法.该方法首先把机器人的执行任务分割成序列化的执行过程,然后通过对比执行过程中累积观察数据的对数似然函数值的大小实现执行过程的识别. 在此基础上,根据正常执行过程训练得到的对数似然函数值的梯度阈值,实现了机器人执行过程中的实时异常监测. 实验结果表明,该方法能有效地实现基于多模态时间序列建模的机器人安全监控. |
关键词: 多模态时间序列 安全监控 执行过程识别 异常监测 层级狄利克雷过程隐马尔可夫模型 梯度阈值 |
DOI:10.11918/201809143 |
分类号:TH375 |
文献标识码:A |
基金项目:广东省联合基金重点项目(U1401240);广东省前沿与关键技术创新专项资金(2014B2,5B010917003, 2017B050506008);国家国际科技合作专项项目(2015DFA11700) |
|
Multimodal time series modeling-based robot safety surveillance |
WU Hongmin1,2,ZHANG Guoying1,GUAN Yisheng1,JUAN Rojas1
|
(1.School of Electromechanical Engineering, Guangdong University of Technology, Guangzhou 510006, China; 2.Guangdong Key Laboratory of Modern Control Technology, Guangdong Institute of Intelligent Manufacturing, Guangzhou 510070, China)
|
Abstract: |
In this paper, considering the problem of multimodal time series modeling based robot safety surveillance, we present a fast, robust, and versatile measure for executing process identification and anomaly detection through Hierarchical Dirichlet Process Hidden Markov Model (HDPHMM). To effectively improve the robot safety surveillance, first the complex manipulation task into sequences of executing processes was decomposed and then the process identification could be achieved by comparing the log-likelihood value of cumulative observations during robot manipulation. After the process identification, the anomaly detection of each process could also be implemented by discriminating anomalies by the gradient of log-likelihood thresholding from the normal training executions. |
Key words: multimodal time series safety surveillance process identification anomaly detection HDPHMM gradient threshold |
|
|
|
|