引用本文: | 朱光轩,张庆松,刘人太,张连震,郭焱旭.基于PSO-LSSVM的砂层可注性预测模型及其敏感性分析[J].哈尔滨工业大学学报,2020,52(11):175.DOI:10.11918/201906047 |
| ZHU Guangxuan,ZHANG Qingsong,LIU Rentai,ZHANG Lianzhen,GUO Yanxu.Groutability prediction in sand stratum using PSO-LSSVM and its sensitivity analysis[J].Journal of Harbin Institute of Technology,2020,52(11):175.DOI:10.11918/201906047 |
|
|
|
本文已被:浏览 1206次 下载 849次 |
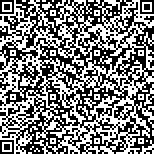 码上扫一扫! |
|
基于PSO-LSSVM的砂层可注性预测模型及其敏感性分析 |
朱光轩1,2,张庆松1,刘人太1,张连震3,郭焱旭1
|
(1. 山东大学 岩土与结构工程研究中心,济南 250061; 2.纽卡斯尔大学 工程与建筑环境学院,澳大利亚 纽卡斯尔; 3.中国石油大学(华东) 储运与建筑工程学院,山东 青岛 266580)
|
|
摘要: |
现有砂层可注性评价方法多为分类评价,且分类标准不一,不利于实际工程应用.为此,采用粒子群算法(PSO)对最小二乘支持向量机(LSSVM)进行优化,提出可注性量化预测模型.选取水泥浆液水灰比RWC、相对密实度Dr、细颗粒(直径<0.075 mm)含量θ、砂层特征粒径D10和D15为控制变量,开展129组可注性室内试验,以每组试验浆液扩散距离作为可注性量化评价指标.基于PSO-LSSVM方法建立砂层可注性与各控制变量间的关系模型,采用傅里叶幅度敏感性测试法(FAST)对可注性影响因素进行全局敏感性分析.结果表明:PSO-LSSVM模型具有较高的预测精度,砂层可注性模型预测值与试验值基本一致,拟合优度R2为0.982;各影响因素对可注性的敏感性排序为:D10>D15>Dr>θ>RWC,其中D10和D15敏感性显著高于Dr、θ和RWC. |
关键词: 注浆 可注性 粒子群算法 最小二乘支持向量机 预测模型 全局敏感性分析 |
DOI:10.11918/201906047 |
分类号:TV543 |
文献标识码:A |
基金项目:国家自然科学基金(3,2); 山东大学基本科研业务费资助项目(2017JC031) |
|
Groutability prediction in sand stratum using PSO-LSSVM and its sensitivity analysis |
ZHU Guangxuan1,2,ZHANG Qingsong1,LIU Rentai1,ZHANG Lianzhen3,GUO Yanxu1
|
(1.Geotechnical and Structural Engineering Research Center, Shandong University, Jinan 250061, China; 2.Faculty of Engineering and Built Environment, University of Newcastle, Callaghan, Australia; 3.College of Pipeline and Civil Engineering, China University of Petroleum, Qingdao 266580, Shandong, China)
|
Abstract: |
Most of the existing evaluation methods for the groutability of sand stratum are classification evaluation methods, and the classification standards are different, which is not conducive to practical engineering application. To solve this problem, particle swarm optimization (PSO) was used to optimize the least squares support vector machine (LSSVM), and a quantitative evaluation model of sand groutability was proposed. With water/cement ratio (RWC), relative density Dr, fine sand content θ (diameter<0.075 mm), and sand characteristic particle size D10 and D15 as control variables, 129 groups of groutability tests were conducted, and grout diffusion distances of each group were measured and used as quantitative evaluation indexes. The relational model between groutability factors and the control variables was obtained by PSO-LSSVM method. Furthermore, the global sensitivity of groutability factors was analyzed by FAST method. Results show that the grout diffusion distance predicted by the PSO-LSSVM model in the test set was close to the test value, the goodness of fit R2 was 0.982, and the prediction model had a high prediction accuracy. The sensitivity sequence of groutability factors was: D10>D15>Dr>θ>RWC, where D10 and D15 were significantly more sensitive than Dr, θ, and RWC. |
Key words: grouting groutability particle swarm optimization (PSO) least squares support vector machine (LSSVM) prediction model global sensitivity analysis |
|
|
|
|