引用本文: | 崔海,余鑫磊,庞继伟,杨珊珊,任南琪,丁杰.采用BP-ANN和改进SVR的进水BOD软测量模型[J].哈尔滨工业大学学报,2022,54(2):59.DOI:10.11918/202111051 |
| CUI Hai,YU Xinlei,PANG Jiwei,YANG Shanshan,REN Nanqi,DING Jie.Influent BOD soft sensing models based on BP-ANN and improved SVR[J].Journal of Harbin Institute of Technology,2022,54(2):59.DOI:10.11918/202111051 |
|
本文已被:浏览 839次 下载 710次 |
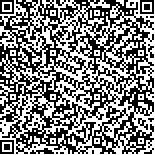 码上扫一扫! |
|
采用BP-ANN和改进SVR的进水BOD软测量模型 |
崔海1,2,余鑫磊1,庞继伟3,杨珊珊1,任南琪1,丁杰1
|
(1.哈尔滨工业大学 环境学院,哈尔滨 150090;2.哈尔滨供水集团有限责任公司,哈尔滨 150010; 3.中国节能环保集团有限公司,北京 100082)
|
|
摘要: |
进水水质条件是研究和优化管理污水处理厂所需的关键要素,及时获取进水水质数据至关重要。针对污水厂关键性水质指标BOD5不易直接检测、滞后强的特点,分别采用BP神经网络(BP-ANN)、网格搜索算法(GS)优化支持向量回归(SVR)、粒子群算法(PSO)优化的SVR和遗传算法(GA)优化的SVR 4种方法,通过利用其他进水指标与进水BOD5的数学关系建立进水BOD5软测量模型,实现进水BOD5快速测定。并以黑龙江某污水厂为研究对象,比较4种机器学习模型的性能,找寻适合进水BOD5预测的软测量方法。结果表明,基于SVR的软测量模型预测结果优于基于BP-ANN的软测量模型,而且采用GA优化的SVR模型精度最高。为实现污水厂进水BOD5的实时监测和污水厂的便捷管理提供了参考依据。 |
关键词: 生化需氧量 BP神经网络 支持向量回归 遗传算法 软测量 |
DOI:10.11918/202111051 |
分类号:X703 |
文献标识码:A |
基金项目:国家重点研发计划项目(2019YFD1100204); 国家自然科学基金面上项目(52170073) |
|
Influent BOD soft sensing models based on BP-ANN and improved SVR |
CUI Hai1,2,YU Xinlei1,PANG Jiwei3,YANG Shanshan1,REN Nanqi1,DING Jie1
|
(1. School of Environment, Harbin Institute of Technology, Harbin 150090, China; 2. Harbin Water Supply Group Co., Ltd., Harbin 150010, China; 3. China Energy Conservation and Environmental Protection Group, Beijing 100082, China)
|
Abstract: |
Influent water quality conditions are the key elements required to investigate and optimize the management of sewage treatment plants, and timely acquisition of influent water quality data is of vital importance. In view of the fact that five-day biochemical oxygen demand (BOD5), a key water quality indicator of sewage plants, is difficult to directly detect and has strong hysteresis, four methods including the back-propagation artificial neural network (BP-ANN), grid search algorithm (GS) optimized support vector regression (SVR), particle swarm optimization (PSO) improved SVR, and genetic algorithm (GA) improved SVR were adopted to establish soft sensing models of influent BOD5 by using the mathematical relationship between BOD5 and other influent parameters to achieve the rapid determination of influent BOD5. A sewage plant in Heilongjiang province was taken as the research object, and the performance of the four machine learning models was compared to find a soft sensing method suitable for the prediction of influent BOD5. Results show that the prediction results of the soft sensing model based on SVR were better than that based on BP-ANN, and the GA optimized SVR model had the highest accuracy, which provides reference for the real-time monitoring of BOD5 and convenient management of sewage treatment plants. |
Key words: biochemical oxygen demand back-propagation neural network support vector regression genetic algorithm soft sensing |