引用本文: | 魏政磊,董康生,段耀泽,陈蔚,岑飞,周欢.融合分割点检测的在线机动轨迹识别方法[J].哈尔滨工业大学学报,2024,56(12):20.DOI:10.11918/202306020 |
| WEI Zhenglei,DONG Kangsheng,DUAN Yaoze,CHEN Wei,CEN Fei,ZHOU Huan.An online maneuver trajectory recognition method based on segmentation point detection[J].Journal of Harbin Institute of Technology,2024,56(12):20.DOI:10.11918/202306020 |
|
|
|
本文已被:浏览 128次 下载 130次 |
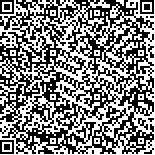 码上扫一扫! |
|
融合分割点检测的在线机动轨迹识别方法 |
魏政磊1,董康生1,段耀泽2,4,陈蔚3,岑飞1,周欢4
|
(1.中国空气动力研究与发展中心, 四川 绵阳 621000; 2.中国人民解放军93642部队, 河北 唐山 063000; 3.中国人民解放军94636部队, 浙江 湖州 313112; 4.空军工程大学 航空工程学院, 西安 710038)
|
|
摘要: |
为提高飞机机动识别方法精准度,提升识别过程的实时性,考虑到战术机动轨迹的动态性以及时序性,融合机动轨迹分割点检测方法,提出一种基于马氏距离度量动态时间规整神经网络的在线机动轨迹识别方法。首先,为防止训练的分割识别模型过拟合,通过提取机动轨迹特征,将机动轨迹飞行参数转化为机动轨迹特征参数,同时构建了包括21种机动轨迹单元的机动库;然后,为快速分割机动轨迹单元,结合支持向量机和马氏距离,提出了一种基于马氏距离支持向量机的机动轨迹分割点检测方法;其次,为提高机动轨迹单元识别精度,结合基于改进马氏距离的动态时间规整与卷积神经网络,提出一种基于马氏距离度量动态时间规整神经网络的机动轨迹单元识别方法;最后,通过融合分割点检测模型与轨迹单元识别模型,构建在线机动轨迹识别平台,并利用3种机动轨迹工况数据对该方法进行仿真分析。仿真结果表明,提出的方法能实时检测飞机机动轨迹单元分割点,且在分割检测的准确率达到97.0%;与其他机动轨迹识别方法相比较,本研究提出的方法不仅满足实时性,且具有较高的识别精度,达到90%以上,从而验证了本研究提出的在线机动识别模型的有效性与实时性。 |
关键词: 在线机动轨迹识别 机动轨迹单元 马氏距离度量 支持向量机 动态时间规整神经网络 |
DOI:10.11918/202306020 |
分类号:V279 |
文献标识码:A |
基金项目:国家自然科学基金(51778186) |
|
An online maneuver trajectory recognition method based on segmentation point detection |
WEI Zhenglei1,DONG Kangsheng1,DUAN Yaoze2,4,CHEN Wei3,CEN Fei1,ZHOU Huan4
|
(1.China Aerodynamics Research and Development Center, Mianyang 621000, Sichuan, China; 2.Unit 93642 of Chinese People’s Liberation Army, Tangshan 063000, Hebei, China; 3.Unit 94636 of Chinese People’s Liberation Army, Huzhou 313112, Zhejiang, China; 4.College of Aeronautics, Air Force Engineering University, Xi’an 710038, China)
|
Abstract: |
To enhance the precision of aircraft maneuver recognition method and improve the real-time of the recognition process, considering the dynamic and temporal nature of tactical maneuver trajectory, through fusing maneuver trajectory segmentation point detection method, an online maneuver trajectory recognition method based on the Mahalanobis distance-based dynamic time warping network is proposed. Firstly, in order to prevent the trained segmentation recognition model from overfitting, the flight parameters of the maneuver trajectory are converted into the maneuver trajectory feature parameters by extracting the maneuver trajectory features, and the maneuver library including 21 maneuver trajectory units is constructed. Secondly, in order to quickly split the maneuver trajectory units, a method is introduced that combines support vector machines and Mahalanobis distance:a maneuver trajectory segmentation point detection method based on Mahalanobis Distance Support Vector Machine. Then, in order to improve the accuracy of maneuver trajectory unit recognition, a method is proposed that combines dynamic time warping based on improved Mahalanobis distance and convolutional neural networks:a maneuver trajectory unit recognition method based on Mahalanobis distance measurement in dynamic time warping neural networks. Finally, by fusing the segmentation point detection model and the trajectory unit recognition model, an online maneuvering trajectory recognition platform is constructed, and the simulation analysis using three maneuver trajectory conditions data is carried out. The experimental results show that the proposed method can detect the segmentation point of the aircraft unit in real time, and the accuracy of the segmentation detection can reach 97.0%. Compared with other maneuver trajectory recognition methods, the proposed method not only meets real-time requirements, but also has high recognition accuracy exceeding 90%. The results validate the effectiveness and real-time performance of the online maneuver recognition model proposed in this research. |
Key words: online maneuver trajectory recognition maneuver trajectory units Mahalanobis distance metric support vector machine(SVM) dynamic time warping network |
|
|
|
|