引用本文: | 陶攀,付忠良,朱锴,王莉莉.空间金字塔分解的深度可视化方法[J].哈尔滨工业大学学报,2017,49(11):60.DOI:10.11918/j.issn.0367-6234.201612087 |
| TAO Pan,FU Zhongliang,ZHU Kai,WANG Lili.Deepvisualization based on the spatial pyramid decomposition[J].Journal of Harbin Institute of Technology,2017,49(11):60.DOI:10.11918/j.issn.0367-6234.201612087 |
|
本文已被:浏览 1860次 下载 1275次 |
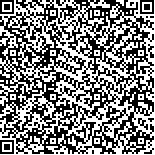 码上扫一扫! |
|
空间金字塔分解的深度可视化方法 |
陶攀1,2,付忠良1,2,朱锴1,2,王莉莉1,2
|
(1.中国科学院 成都计算机应用研究所, 成都 610041; 2.中国科学院大学, 北京 100049)
|
|
摘要: |
针对基于深度卷积神经网络的图像分类模型的可解释性问题,通过评估模型特征空间的潜在可表示性,提出一种用于改善理解模型特征空间的可视化方法.给定任何已训练的深度卷积网络模型,所提出的方法在依据原输入图像使得模型类别得分激活最大化时,首先对反向传播的梯度进行归一化操作,然后采用带动量的随机梯度上升训练策略,反向回传修改原输入图像.引入了通过激活最大化获得的图像可解释性的正则化方法,常规正则化技术不能主动调整模型特征空间的潜在可表示性,结合现有正则化方法提出空间金字塔分解方法,利用构建多层拉普拉斯金字塔主动提升目标图像特征空间的低频分量,结合多层高斯金字塔调整其特征空间的高频分量得到较优可视化效果.通过限制可视化区域,提出利用类别显著性激活图技术加以压制上下文无关信息,可进一步改善可视化效果.对模型学习到的不同类别和卷积层中单独的神经元进行合成可视化实验,实验结果表明提出的方法在不同的深度模型和不同的可视化任务中均能取得较优的可视化效果.
|
关键词: 深度可视化 金字塔分解 激活最大化 卷积神经网络 激活图 |
DOI:10.11918/j.issn.0367-6234.201612087 |
分类号:TP391.41 |
文献标识码:A |
基金项目:中国科学院西部之光人才培养计划项目 |
|
Deepvisualization based on the spatial pyramid decomposition |
TAO Pan1,2,FU Zhongliang1,2,ZHU Kai 1,2,WANG Lili1,2
|
(1. Chengdu Institute of Computer Application, Chinese Academy of Sciences, Chengdu 610041, China; 2. University of Chinese Academy of Sciences, Beijing 100049, China)
|
Abstract: |
Focusing on the interpretability problems of image classification models based on deep convolutional neural network, a visualization method for improving the feature space of model is proposed by evaluating the potential expressiveness of model feature space. Given any pre-trained deep model, firstly the method generates an image by the normalized operation of the gradient in the back propagation, which maximizes activation the class score, and then uses the momentum of the stochastic gradient descent training strategy for back propagation to the original input image. The conventional regularization technique cannot adjust the feature space of the model. Therefore, the spatial pyramid decomposition method is proposed on the basis of the existing regularization method. By constructing the multi-layer Laplacian spatial pyramid, the low frequency component of the target image feature space is promoted, combined with multi-layer Gaussian spatial pyramid to adjust the high-frequency components of its feature space to obtain a better visualization effect. By limiting the region of visualization, it is proposed to use the class activation map to suppress the context-free information, which can further improve the visualization effect. The visualization experiments are performed on the different classes of the model and the individual neurons of the convolution layer. Results show that the proposed method can achieve better visualization effect in different depth models and different visualization tasks.
|
Key words: deep visualization pyramid decomposition maximize activation convolutional neural network activation map |