引用本文: | 于烨,黄默,段涛,王长元,胡锐.粒子群优化加权灰色回归组合的卫星钟差预报[J].哈尔滨工业大学学报,2020,52(10):144.DOI:10.11918/201911175 |
| YU Ye,HUANG Mo,DUAN Tao,WANG Changyuan,HU Rui.Satellite clock bias prediction based on particle swarm optimization and weighted grey regression combined model[J].Journal of Harbin Institute of Technology,2020,52(10):144.DOI:10.11918/201911175 |
|
|
|
本文已被:浏览 840次 下载 791次 |
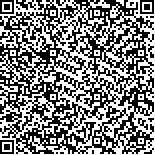 码上扫一扫! |
|
粒子群优化加权灰色回归组合的卫星钟差预报 |
于烨1,2,黄默1,3,段涛1,王长元1,胡锐1
|
(1.中国科学院 微电子研究所,北京 100029; 2.中国科学院大学,北京 100049; 3.中国科学院大学 微电子学院,北京 100049)
|
|
摘要: |
为了提高卫星钟差预报的精度和稳定度,提出了一种基于粒子群算法优化指数函数和线性函数逼近的加权灰色回归组合的自适应卫星钟差预报方法.该方法首先在建模之前考虑到卫星钟差钟跳频繁的现象,采用中位数法探测钟跳数据并将其剔除后,采用分段线性插值法将缺失的钟差数据补齐;然后考虑到卫星钟差存在系统噪声,采用三点平滑法对钟差数据进行平滑处理后,建立了以指数函数和线性函数逼近加权灰色回归组合的卫星钟差预报模型.针对模型中的精度递增因子难以确定的问题,采用粒子群优化算法对精度递增因子进行自适应寻优.最后,采用IGS服务器上发布的事后精密卫星钟差产品,并结合4种典型变化趋势的卫星钟差进行了6 h的预报试验.试验结果表明:该方法的预报性能明显优于其他几种常用模型,其6 h的平均预报精度(RMS)和稳定度(Range)相对于常用的二次多项式预报模型、灰色预报模型、修正指数曲线法预报模型和自回归滑动平均预报模型分别提高了79.10%、44.00%、80.70%、32.30%和63.10%、29.80%、77.60%、26.30%. |
关键词: 卫星钟差预报 系统噪声 三点平滑法 加权灰色回归法 精度递增因子 粒子群优化算法 |
DOI:10.11918/201911175 |
分类号:TN967.1 |
文献标识码:A |
基金项目:中国博士后科研基金项目(2019M650801) |
|
Satellite clock bias prediction based on particle swarm optimization and weighted grey regression combined model |
YU Ye1,2,HUANG Mo1,3,DUAN Tao1,WANG Changyuan1,HU Rui1
|
(1.Institute of Microelectronics, Chinese Academy of Sciences, Beijing 100029, China; 2.University of Chinese Academy of Sciences, Beijing 100049, China; 3.School of Microelectronics, University of Chinese Academy of Sciences, Beijing 100049, China)
|
Abstract: |
To improve the accuracy and stability of satellite clock bias (SCB) prediction, an adaptive SCB prediction method was proposed based on the combination of particle swarm optimization (PSO) and weighted grey regression with index function and linear function approximation. First, considering the phenomenon of frequent SCB clock jumps, the clock jump data was detected and excluded through median absolute deviation (MAD) before modeling, and the piecewise linear interpolation method was used to complement the missing clock bias data. Then, in order to deal with the system noise of the SCB data, the three-point smoothing method was used to smooth the clock bias data. After the processing, an SCB prediction model based on the combination of exponential function and linear function approximation for weighted grey regression was established. Aiming at the problem that the increasing precision factor in the model was difficult to determine, a PSO algorithm was used to adaptively optimize the accuracy increasing factor. Finally, a 6-hour forecast test was performed by adopting the post-accurate SCB product released on the IGS server and combining with four typical trends. Experimental results show that the prediction performance of the proposed method was significantly better than those of other commonly used models. Compared with the quadratic polynomial prediction model (QPM), grey prediction model (GM (1,1)), modified exponential curve method (MECM), and autoregressive moving average model (ARMA), the 6-hour average forecast accuracy (RMS) and stability (Range) of the proposed method were increased by 79.10%, 44.00%, 80.70%, 32.30%, and 63.10%, 29.80%, 77.60%, 26.30%, respectively. |
Key words: satellite clock bias prediction system noise three-point smoothing method weighted grey regression method increasing precision factor particle swarm optimization algorithm |
|
|
|
|