引用本文: | 王殿伟,方浩宇,刘颖,伍世虔,谢永军,宋海军.一种基于改进RT-MDNet的全景视频目标跟踪算法[J].哈尔滨工业大学学报,2020,52(10):152.DOI:10.11918/201910175 |
| WANG Dianwei,FANG Haoyu,LIU Ying,WU Shiqian,XIE Yongjun,SONG Haijun.Improved RT-MDNet for panoramic video target tracking[J].Journal of Harbin Institute of Technology,2020,52(10):152.DOI:10.11918/201910175 |
|
|
|
本文已被:浏览 891次 下载 810次 |
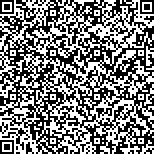 码上扫一扫! |
|
一种基于改进RT-MDNet的全景视频目标跟踪算法 |
王殿伟1,方浩宇1,刘颖1,伍世虔2,谢永军3,宋海军3
|
(1.西安邮电大学 通信与信息工程学院,西安 710121; 2.武汉科技大学 信息科学与工程学院,武汉 430081; 3.中国科学院 西安光学精密机械研究所,西安 710119)
|
|
摘要: |
为了解决全景视频目标跟踪过程中,由于光照条件变化、相似背景干扰、目标运动时产生的形变和尺度变化等因素的影响,在跟踪中会出现目标漂移、目标丢失等情况,进而导致目标跟踪算法成功率低,鲁棒性差等问题,提出一种基于长短期记忆网络和改进Real-Time MDNet网络的全景视频目标跟踪方法.算法首先采用浅层卷积神经网络提取特征,并利用自适应的RoIAlign减少特征提取过程中的像素损耗,而后运用目标特征在线更新最后一个全连接层的权重,在全连接层中实现前景背景分离并提取出目标区域,然后通过长短期记忆网络自适应地选取目标框的尺度,最终输出目标位置信息.实验结果表明:单目算法应用在全景数据集时,难以适应全景中的尺度变化和背景变化,改进算法利用3层长短期记忆网络构建的尺度预测模块,可以有效地应对全景数据存在的尺度变化和目标形变问题,在保持较好的跟踪精度的同时,可以有效地应对目标跟踪中出现的小目标、目标遮挡、多目标交叉运动的情况,获得更好的视觉效果和更高的重叠率得分. |
关键词: 目标跟踪 深度学习 全景视频 长短期记忆网络 RT-MDNet |
DOI:10.11918/201910175 |
分类号:TP391.41;TP183 |
文献标识码:A |
基金项目:公安部科技强警基础研究专项项目(2019GABJC42);陕西省自然科学基础研究计划(创新创业 “双导师”)研究项目(2018JM6118);西安邮电大学研究生创新基金(CXJJLY2018033) |
|
Improved RT-MDNet for panoramic video target tracking |
WANG Dianwei1,FANG Haoyu1,LIU Ying1,WU Shiqian2,XIE Yongjun3,SONG Haijun3
|
(1.School of Communications and Information Engineering, Xi’an University of Posts and Telecommunications, Xi’an 710121, China; 2.School of Information Science and Engineering, Wuhan University of Science and Technology, Wuhan 430081, China; 3.Xi’an Institute of Optics and Precision Mechanics, Chinese Academy of Sciences, Xi’an 710119, China)
|
Abstract: |
In the process of panoramic video target tracking, the target deformation and scale changes caused by light change, interference of similar background, and object moving may result in target drift or missing, leading to low success rate and poor robustness. To address these issues, a target tracking method based on long short-term memory (LSTM) network and improved Real-Time MDNet (RT-MDNet) network was proposed. First, shallow convolution neural network was utilized to extract features, and adaptive RoIAlign was adopted to reduce pixel loss in the convolution process. Then, the weight of the last layer of the full connection layers was updated online by utilizing the target features to achieve foreground background separation and extract the target area. Lastly, the scale of the target box was selected adaptively by means of LSTM, and the target position information was thus obtained. Experimental results show that monocular vision algorithm could hardly adapt to the scale change and background change when applied in panoramic dataset, while the proposed method that utilizes 3-layer LSTM network to construct scale prediction module could effectively solve these problems. The algorithm can efficiently deal with the situations of small target, target occlusion, and cross motion of multiple targets in target tracking while maintaining accuracy, achieving better visual effect and higher overlap rate score. |
Key words: target tracking deep learning panoramic video LSTM RT-MDNet |
|
|
|
|