引用本文: | 周洲,焦文玲,任乐梅,田兴浩.蚁群算法分配权重的燃气日负荷组合预测模型[J].哈尔滨工业大学学报,2021,53(6):177.DOI:10.11918/201911084 |
| ZHOU Zhou,JIAO Wenling,REN Lemei,TIAN Xinghao.Combined forecasting model of gas daily load based on weight distribution of ant colony algorithm[J].Journal of Harbin Institute of Technology,2021,53(6):177.DOI:10.11918/201911084 |
|
|
|
本文已被:浏览 792次 下载 739次 |
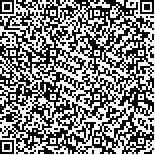 码上扫一扫! |
|
蚁群算法分配权重的燃气日负荷组合预测模型 |
周洲1,2,焦文玲1,2,任乐梅1,2,田兴浩1,2
|
(1.哈尔滨工业大学 建筑学院,哈尔滨 150090; 2.寒地城乡人居环境科学与技术工业和信息化部重点实验室 (哈尔滨工业大学),哈尔滨 150090)
|
|
摘要: |
为适应城镇燃气日负荷随机性和多变性的特点,克服特定时刻单一负荷预测模型存在实际应用局限性的问题,将5种评价准则用于组合预测前剔除冗余模型,提出了一种建立变全重组合预测模型的方法,通过蚁群算法确定分配权重的组合预测模型,使得在一个时段上的燃气日负荷预测精度好于各单一模型.首先对包含诸多随机和模糊等不确定因素的城镇燃气日负荷时变系统和各预测模型特点进行分析;然后确定岭回归分析(Ridge)、差分自回归积分滑动平均模型(ARIMA)、支持向量机回归(SVR)、极端梯度提升树(XGB)共4类单项日负荷预测模型,结合城镇燃气日负荷和模型的特点,分别给出每个模型各项参数的设置和模型的输入向量;用平均相对误差、均方根误差、灰色关联度、相关系数、Theil不等系数为评价准则计算出的综合评价指标剔除冗余模型,最后建立了蚁群算法权重分配的组合预测模型. 预测实例表明,蚁群算法分配权重的燃气日负荷组合预测模型长期的综合预测效果要优于任意单项模型,相比于单一模型而言,组合预测模型的稳定性和容错率更高,具备较强的泛化能力. |
关键词: 城镇燃气日负荷 组合预测 岭回归 差分自回归积分滑动平均 支持向量机回归 极端梯度提升树 蚁群算法 |
DOI:10.11918/201911084 |
分类号:TU996 |
文献标识码:A |
基金项目: |
|
Combined forecasting model of gas daily load based on weight distribution of ant colony algorithm |
ZHOU Zhou1,2,JIAO Wenling1,2,REN Lemei1,2,TIAN Xinghao1,2
|
(1.School of Architecture, Harbin Institute of Technology, Harbin 150090, China; 2.Key Laboratory of Cold Region Urban and Rural Human Settlement Environment Science and Technology (Harbin Institute of Technology), Ministry of Industry and Information Technology, Harbin 150090, China)
|
Abstract: |
Urban gas daily load has the characteristics of randomness and variability, and single load forecasting models have certain limitations in practical applications, especially for specific time periods. To tackle such problem, five evaluation criteria were used to eliminate redundant model before combination forecasting, and a method of constructing variable weight combined forecasting model was proposed. The distribution weights of the combined forecasting model were determined by ant colony algorithm, so that the precision of the combined forecasting model was better than single models in a certain time period. First, the time-varying system of urban gas daily load and the characteristics of each forecasting model were analyzed, which contains many random and fuzzy uncertainties. Then, four kinds of single daily load forecasting models were determined, including ridge regression (Ridge), differential autoregressive integral moving average model (ARIMA), support vector machine regression (SVR), and extreme gradient lifting tree (XGB). According to the characteristics of urban gas daily load model, the parameters of each model and the input vector of the model were given. The redundant model was eliminated by using the comprehensive evaluation indexes calculated by the average relative error, root mean square error, grey correlation degree, correlation coefficient, and Theil unequal coefficient as the evaluation criteria. Finally, based on the ant colony algorithm, a weight distribution combination forecasting model was developed. Study results show that the long-term comprehensive prediction effect of the proposed model was superior to that of any single model. Compared with a single model, the combined forecasting model had higher stability and fault tolerance rate, and stronger generalization ability. |
Key words: urban gas daily load combined forecasting ridge regression differential autoregressive integral moving average support vector machine regression extreme gradient lifting tree ant colony algorithm |
|
|
|
|