引用本文: | 费鸿博,吴伟官,李平,曹毅.基于梅尔频谱分离和LSCNet的声学场景分类方法[J].哈尔滨工业大学学报,2022,54(5):124.DOI:10.11918/202104081 |
| FEI Hongbo,WU Weiguan,LI Ping,CAO Yi.Acoustic scene classification method based on Mel-spectrogram separation and LSCNet[J].Journal of Harbin Institute of Technology,2022,54(5):124.DOI:10.11918/202104081 |
|
|
|
本文已被:浏览 744次 下载 1059次 |
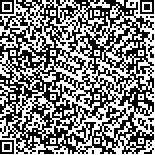 码上扫一扫! |
|
基于梅尔频谱分离和LSCNet的声学场景分类方法 |
费鸿博1,2,吴伟官1,2,李平1,2,曹毅1,2
|
(1.江南大学 机械工程学院,江苏 无锡 214122; 2.江苏省食品先进制造装备技术重点实验室(江南大学),江苏 无锡 214122)
|
|
摘要: |
针对现有频谱分离方法进行声学场景分类研究时其分类准确率不高的问题,提出了一种基于梅尔频谱分离和长距离自校正卷积神经网络(long-distance self-calibration convolutional neural network,LSCNet)的声学场景分类方法。首先,介绍了频谱的谐波打击源分离原理,提出了一种梅尔频谱分离算法,将梅尔频谱分离出谐波分量、打击源分量和残差分量;然后,结合自校正神经网络和残差增强机制,提出了一种长距离自校正卷积神经网络;该模型采用频域自校正算法以及长距离增强机制来保留特征图原始信息,通过残差增强机制和通道注意力增强机制加强了深层特征与浅层特征间的关联度,且结合多尺度特征融合模块,以进一步提取模型训练中输出层的有效信息,从而提高模型的分类准确率;最后,基于Urbansound8K和ESC-50数据集开展了声学场景分类实验。实验结果表明:梅尔频谱的残差分量能够针对性地减少背景噪音的影响,从而具有更好的分类性能,且LSCNet实现了对特征图中频域信息的关注,其最佳分类准确率分别达到90.1%和88%,验证了该方法的有效性。 |
关键词: 声学场景分类 梅尔频谱分离算法 长距离自校正卷积神经网络 频域自校正算法 多尺度特征融合 |
DOI:10.11918/202104081 |
分类号:TP391.42 |
文献标识码:A |
基金项目:高等学校学科创新引智计划(B18027); 江苏省“六大人才高峰”计划(ZBZZ-012); 江苏省优秀科技创新团队基金(2019SK07) |
|
Acoustic scene classification method based on Mel-spectrogram separation and LSCNet |
FEI Hongbo1,2,WU Weiguan1,2,LI Ping1,2,CAO Yi1,2
|
(1.School of Mechanical Engineering, Jiangnan University, Wuxi 214122, Jiangsu, China;2.Jiangsu Key Laboratory of Advanced Food Manufacturing Equipment and Technology (Jiangnan University), Wuxi 214122, Jiangsu, China)
|
Abstract: |
When the existing spectrogram separation methods are used for acoustic scene classification research, the classification accuracy of these methods is not high. To solve the problem, an acoustic scene classification method based on Mel-spectrogram separation and long-distance self-calibration convolutional neural network (LSCNet) was proposed. Firstly, the working principles of spectrogram harmonic/percussive-source separation were presented. A Mel-spectrogram separation algorithm was proposed, which can separate the Mel-spectrogram into harmonic components, percussive source components, and residual components. Then, LSCNet was designed combining self-calibration convolutional network and residual enhancement mechanism. The model adopts frequency domain self-correction algorithm and long-distance enhancement mechanism to retain the original information of the feature map, strengthens the correlation between deep and shallow features through residual enhancement mechanism and channel attention enhancement mechanism, and combines multi-scale feature fusion module to further extract the effective information of the output layer in model training. Finally, acoustic scene classification experiments were conducted on Urbansound8K and ESC-50 datasets. Experimental results show that the Mel-spectrogram residual components (MSRC) could specifically reduce the influence of background noise, thereby indicating a better classification performance. The LSCNet could realize the attention to the frequency domain information in the feature map, and its best classification accuracy reached 90.1% and 88% respectively, which verified the effectiveness of the proposed method. |
Key words: acoustic scene classification Mel-spectrogram separation algorithm LSCNet frequency domain self-calibration algorithm multi-scale feature fusion |
|
|
|
|