引用本文: | 黄靖,汤宁,文元桥,郭玉滨,朱立夫,肖长诗.多尺度水上船舶目标视觉检测[J].哈尔滨工业大学学报,2024,56(5):103.DOI:10.11918/202201030 |
| HUANG Jing,TANG Ning,WEN Yuanqiao,GUO Yubin,ZHU Lifu,XIAO Changshi.Multi-scale visual detection for waterborne ship targets[J].Journal of Harbin Institute of Technology,2024,56(5):103.DOI:10.11918/202201030 |
|
|
|
本文已被:浏览 1236次 下载 1524次 |
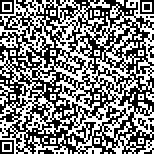 码上扫一扫! |
|
多尺度水上船舶目标视觉检测 |
黄靖1,汤宁1,文元桥2,3,郭玉滨1,朱立夫1,肖长诗2,3
|
(1.武汉理工大学 计算机与人工智能学院,武汉 430063;2.武汉理工大学 国家水运安全工程技术研究中心,武汉 430063; 3.武汉理工大学 智能交通系统研究中心,武汉 430063)
|
|
摘要: |
水上交通场景环境复杂,通过普通光学摄像设备获取的水面图像,面临着视觉目标清晰度低、尺度多样化等问题,使得可见光视觉信号里中、小尺度目标检测相对困难。为服务于各类智慧海事应用,提出了一个旨在提高复杂水域背景下多尺度水上船舶目标检测性能的算法(multi-scale ship object detection,MS-SOD)。该算法基于当前计算机视觉技术中主流的单阶段目标检测模型框架,在其主干网络中嵌入卷积注意力模块,来优化船舶特征提取能力;在多尺度特征融合网络中引入富含细节信息的浅层特征,并使用跨阶段局部残差结构,来优化多尺度船舶特征的融合机制;同时,使用焦点损失函数,来优化模型的学习过程;并设计自适应锚框聚类算法优化先验锚框,以提高多尺度船舶目标检测能力。为验证提出算法的有效性和实效性,在构建较大规模水上船舶目标数据集的基础上,开展了广泛实验验证。结果表明:提出的算法在测试数据集上的检测准确度超过了各主流的对比方法;特别是对于大、中、小各尺度船舶目标的检测精度,相对于主流的YOLOv4算法,提出的算法分别提升了11.3%、6.0%和10.5%。 |
关键词: 多尺度船舶 目标检测 深度学习 注意力机制 特征融合 |
DOI:10.11918/202201030 |
分类号:TP399 |
文献标识码:A |
基金项目:国家自然科学基金(52072287);浙江省科技计划(2021C01010) |
|
Multi-scale visual detection for waterborne ship targets |
HUANG Jing1,TANG Ning1,WEN Yuanqiao2,3,GUO Yubin1,ZHU Lifu1,XIAO Changshi2,3
|
(1.School of Computer Science and Artificial Intelligence, Wuhan University of Technology, Wuhan 430063, China; 2.National Engineering Research Center Water Transport Safety, Wuhan University of Technology, Wuhan 430063, China; 3.Intelligent Transportation Systems Research Center, Wuhan University of Technology, Wuhan 430063, China)
|
Abstract: |
The water transportation environment poses challenges in terms of complexity, making it difficult to achieve clear and diverse visual target detection in water surface images captured by conventional optical cameras. This difficulty is particularly prominent when detecting medium- and small-scale objects in visible light visual signals. For the development of smart maritime applications, we proposed a multi-scale ship object detection (MS-SOD) algorithm to improve the performance of multi-scale ship object detection in complex waters. MS-SOD is built based on the mainstream framework of one-stage object detection models. The convolutional block attention module is embedded into its backbone network to optimize the ability of ship feature extraction. The shallow features with rich detailed information are added to the multi-scale feature fusion network, and cross-stage-partial residual structure is used to enhance the fusion mechanism of multi-scale ship object features. Additionally, a focal loss function is employed to optimize the training process of the model, and an adaptive anchor clustering algorithm is designed to optimize the prior anchor and improve the detection capability for multi-scale ship objects. Extensive experiments are conducted on a self-built large-scale ship object dataset to validate the effectiveness and efficiency of the proposed MS-SOD algorithm. Experimental results show that the accuracy of MS-SOD outperforms various mainstream comparative methods on test dataset. Especially, compared with the YOLOv4 algorithm, the detection accuracy of large-, medium-, and small-scale ship objects improve by 11.3%, 6.0%, and 10.5%, respectively. |
Key words: multi-scale ship object detection deep learning attention mechanism feature fusion |
|
|
|
|