引用本文: | 邓友生,张克钦,李文杰,李龙,彭程谱,姚志刚.卷积神经网络与随机场分析桩梁基础承载力[J].哈尔滨工业大学学报,2024,56(9):124.DOI:10.11918/202306001 |
| DENG Yousheng,ZHANG Keqin,LI Wenjie,LI Long,PENG Chengpu,YAO Zhigang.Convolutional neural network and random field analysis for analyzing the bearing capacity of coupling beam pile foundation[J].Journal of Harbin Institute of Technology,2024,56(9):124.DOI:10.11918/202306001 |
|
|
|
本文已被:浏览 723次 下载 1072次 |
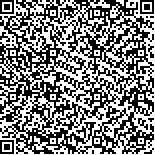 码上扫一扫! |
|
卷积神经网络与随机场分析桩梁基础承载力 |
邓友生1,2,张克钦1,2,李文杰1,2,李龙1,2,彭程谱3,姚志刚1,2
|
(1.西安科技大学 建筑与土木工程学院,西安 710054;2.西安科技大学 桩承结构研究中心, 西安 7100542;3.哈尔滨工业大学 土木工程学院,哈尔滨 150090)
|
|
摘要: |
岩土体的参数在空间上随机分布,为能更好地反应实际工程地质条件,在桩基础承载力研究中考虑土体的不确定性,并建立具有重要工程价值的承载力预测模型,将基于随机场理论的岩土参数空间变异性引入桩梁基础的研究中,采用数值方法建立桩梁基础与群桩基础的二维随机有限元模型分析承载能力,并与模型试验结果验证。随后通过卷积神经网络建立土体参数随机场图像与基础极限承载力之间的模型进行承载力预测,并基于预测模型研究不同参数的影响。结果表明:考虑土体空间变异性的基础承载力与试验结果基本吻合,随机结果均高于确定性分析;随机场下桩梁基础与群桩基础的承载力均为正态分布;采用卷积神经网络建立的基础承载力预测模型精度较高,且可以用于参数分析,基础承载力随着土体参数的增加而增加,随变异系数的增加而下降。随机条件下,桩梁基础的承载力高于群桩基础,可以充分发挥土体强度并抵御参数不确定性带来的承载力损失。 |
关键词: 桩梁基础 空间变异性 随机场理论 卷积神经网络 承载力 |
DOI:10.11918/202306001 |
分类号:TU473.1 |
文献标识码:A |
基金项目:国家自然科学基金(4,8);陕西省自然科学基础研究计划重点项目(2018JZ5012) |
|
Convolutional neural network and random field analysis for analyzing the bearing capacity of coupling beam pile foundation |
DENG Yousheng1,2,ZHANG Keqin1,2,LI Wenjie1,2,LI Long1,2,PENG Chengpu3,YAO Zhigang1,2
|
(1.School of Architecture and Civil Engineering, Xi′an University of Science and Technology, Xi′an 710054, China; 2.Pile-supported Structures Research & Test Center,Xi′an University of Science and Technology, Xi′an 710054, China; 3.School of Civil Engineering, Harbin Institute of Technology, Harbin 150090, China)
|
Abstract: |
The parameters of geotechnical are randomly distributed in space. To better reflect the actual engineering geological conditions, considering the uncertainty of soil in the study of foundation bearing capacity, establishing a bearing capacity prediction model holds significant engineering value. Incorporating spatial variability of geotechnical parameters based on random field theory into the study of coupling beam pile foundation, a two-dimensional random finite element model is established using numerical methods to analyze the bearing capacity of coupling beam pile foundation and pile foundation, validated against model test results. Subsequently, a convolutional neural network is employed to establish a model between the random field images of soil parameters and the ultimate bearing capacity of foundations for bearing capacity prediction, and the impact of different parameters is studied based on the prediction model. The results indicate that considering the spatial variability of soil, the foundation′s bearing capacity is in basic agreement with experimental results, with random results consistently higher than deterministic analysis. Under random conditions, both coupling beam pile and pile foundations exhibit normally distributed bearing capacities. The accuracy of the bearing capacity prediction model established using convolutional neural networks is high and can be utilized for parameter analysis. The bearing capacity of foundations increases with increasing soil parameters and decreases with increasing coefficient of variation. Under random conditions, the bearing capacity of coupling beam pile foundation is higher than that of pile foundation, effectively leveraging soil strength to withstand bearing capacity loss caused by parameter uncertainties. |
Key words: coupling beam pile foundation spatial variability random field theory convolutional neural network load bearing capacity |
|
|
|
|